We have seen a significant shift in science and technology through the evolution of AI. The transformation did not just happen within a night, it took years. It has evolved from a theoretical concept to a practical technology. With the rapidly shaping technological map, the innovation of AI has created a big future. It has changed the way how we all consider our relationship with machines.
In this article, we have tried to incorporate everything about the evolution of AI, the key moments, and examples, and its present and future implications.
What is AI?
In computer science, Artificial Intelligence (AI) is an individual field that gives machines the ability to perform tasks requiring human intelligence. AI uses algorithms, data and computational capability for systems to sense. and interpret language understanding (spoken or written), global insight etc.
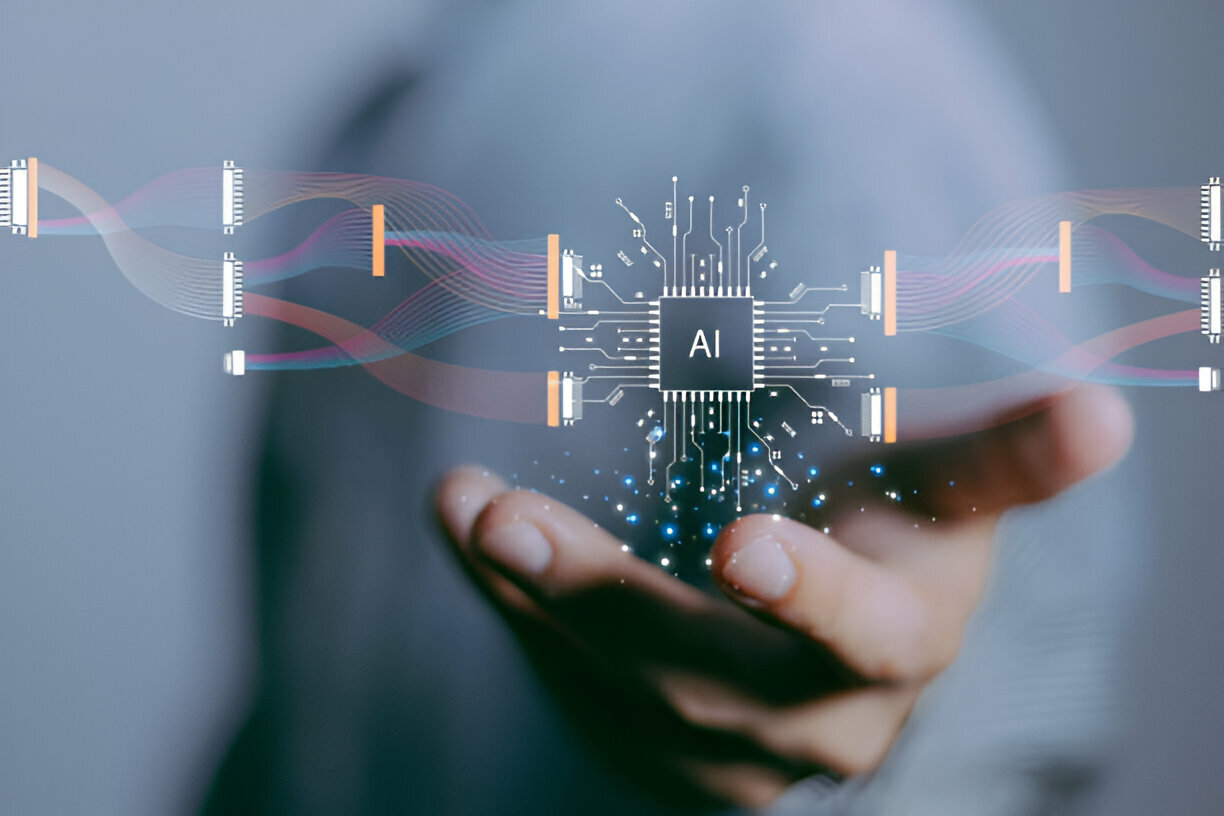
AI can spot patterns, interpret natural language (written or spoken), and process unstructured information from large volumes of data to the point where it can take educated actions. It learns from his past experiences, shares the behaviour with parallel instances and solves large-scale problems.
The Roots of Artificial Intelligence
Intelligent machines have been in the realm of imagination before modern computing. Even in the search for the roots of AI, we came across myths and stories. Because, it is believed that the birth of AI, the need for AI has come through myths. Just as ancient myths like the Greek story of Talos, a mechanical man with human attributes.
The conceptual backbone of AI as we know it was founded by these philosophers (René Descartes, Thomas Hobbes), problematizing the concepts of thought and intelligence.
The formal study of AI started in the middle of the last century. In 1950, the British mathematician Alan Turing (one of the founders of AI) described the so-called Turing Test to judge a machine’s capacity to exhibit human-like intelligence.
In the same era, other pioneers such as John McCarthy, Marvin Minsky, and Claude Shannon initiated their findings about AI at the 1956 Dartmouth Conference.
The Era of 1900 – Groundwork Before The Evolution of AI
The 1900s went on to feature increased artistic experiments on artificial humans. A concept that spread greatly through literature, art and theatre; sparked the ingrain scientific interest. Czech playwright Karel Čapek first used the word “robot” in his 1921 play Rossum’s Universal Robots. During this period, we saw initial efforts in the pursuit of artificial reasoning (that eventually resulted in legendary milestones such as Gakutensoku, the Japanese professor of the robot mission and “Introduction of Robot to Education,”1929 or Edmund Berkley, Giant Brains in Advance), too.
The Birth of AI (1950-1956)
The middle of the 20th century was a crossroads for AI. Alan Turing, one of the leading lights of computing, came up with the Turing Test in his 1950 paper “Computer Machinery and Intelligence” to measure whether a machine “thought”. In 1955 the term artificial intelligence (AI) was coined at a Dartmouth Conference, where John McCarthy was the designated leader who established AI as an independent discipline of study.
Expansion in times of adversity: 1957–1979
AI Research started to develop with some of the most important achievements the development of LISP (invented as a high-level language for AI) & the first industrial robot Unimate. This era also spawned the creation of some of the earliest expert systems, as well as first-generation autonomous vehicles. However, progress started to very slow in the 1970s as government interest went out and funding dropped.
The AI Boom and Winter: 1980-1993
The 1980s brought the “AI boom” with governments and industries pouring money into AI projects. Central among these was Japan’s Fifth Generation Computer Project which sought to build human-level reasoning machines. However, unrealistic expectations and short-term gains versus long-term vision resulted in the first AI “Winter,” during the early 1990s. It was a time of decreased investment and interest in AI projects.
The Challenges and Limitations
While this was the time when much progress was made, AI still had some big problems and limitations. The main limitation was that early computers had such little processing power and limited data storage. These limitations prevent the capability to evolve and prepare intricate AI algorithms, minifying the reach, and efficacy from which an AI system can attain.
Another issue was with real-world challenges of tasks followed by complexity and variability. For the most part, early AI systems could not operate well in uncontrolled environments due to a lack of robustness and an inability to cope with real-world unpredictability and diversity.
The Emergence of AI Autonomous Agent: 1993–2011
AI fell into disuse again only to be revived in the 1990s with AI research having an intermediary success in practical applications. In 1997, IBM’s Deep Blue beat chess Grandmaster Garry Kasparov; innovations like the Roomba led AI into consumer markets At this time we saw great advances in speech recognition robotics and autonomous navigation (see Mars rovers)
Major Achievements
Image/Speech Recognition Benchmarks
Deep learning has pushed the state-of-the-art in image and speech recognition to achieve results far beyond anything we humans are capable of:
Image Recognition: Convolutional neural networks (CNNs) have enabled huge improvements in recognising images (e.g face recognition, object detection, medical image analysis etc).
AlexNet, VGGNet and ResNet – have pushed the accuracy of these models to excellent levels on some benchmark datasets.
Speech Recognition – Recurrent neural networks and especially, long short-term memory (LSTM) networks have been applied to speech recognition systems, allowing for accurate transcribing and voice command recognition. Amazon’s Alexa and Apple’s Siri are powered by deep learning models in virtual assistants.
Priming examples include DeepMind’s Google AlphaGo (2016) defeating world champion Lee Sedol (2016) in the board game of Go; one of the rarely “solved” games postcluding chess.
AlphaGo used deep reinforcement learning (functioning as neural networks in Go levels shuffle exploration and decision-making on board play positions.
The win showed how deep learning can handle difficult, high-level strategic problems — things that had previously been considered too computationally difficult for AI.
Impact on different sectors
Impact On Driver’s Industry, Healthcare, Finance etc: Deep learning algorithms have changed the world of Dhwani in many ways driving innovation and increasing the efficiency of services provided in diverse areas,
Deep Learning: When the medical images are the data, deep learning models are used for medical image analysis (diagnosis) / disease discovery / personalized medicine.
For instance, AI can even read medical X-rays and MRIs with a high degree of accuracy to detect abnormalities that help in the early diagnosis and treatment of diseases.
AI in the age of today: 2012 – Now
In the last decade, AI has integrated into most aspects of life. The study on deep neural networks came with achievement and state-of-the-art results. A successful drive includes image recognition, natural language processing, and machine translation.
With AI deep learning and big data analytics, humans have their AI companions under touch. AI started to transform our lives, ranging from virtual assistants like Siri to GPT-3 (a language model that can write what looks like human text). From personalized recommendations on Netflix to self-driving cars and robotic citizens like Sophia. AI today powers applications.
Current Challenges
Ethical Questions: With the wider spread of AI technologies, the following ethical issues need to be cleared:
Bias – AI models often learn the bias of the training data, which leads to unfair and biased output Ensuring fairness, as well as prohibiting bias in AI system is fundamental to trust and preventing harm.
Privacy – Large-scale personal data collection and use comes with a lot of privacy concerns. Privacy of the user and adherence to privacy regulations are there to keep public trust in AI technologies.
Transparency – Most AI models (especially deep learning models) are opaque, or “black boxes” that are challenging to interpret and explain their decision processes. Creating more transparent and interpretable AI systems is fundamental to the goal of transparency and auditability.
Technical Challenges – There are a few remaining technical hurdles behind the viability and deployment of AI systems.
Continuum of Interpretability – AI models lie on a spectrum from high to low interpretation, due in large part to AIAIMA’s Generalization being the key topic and ability of an AI system (or a human) to learn from limited data, generalize patterns, and perform well on unseen but related problems across given environments.
Explanation capacity – For humans to be able to understand and trust an AI model, we need to find ways to make it easier to understand the output of the AI model.
AI models must be robust – That is to say, they should work generally well in all applications, withstand adversarial attacks and be okay with the unexpected stuff in real-world settings.
The Future of AI – Endless Possibilities
Some experts think that the path of AI could be “embodied AI” or the “embodied cognition meeting”. It means we believe that in the future, AI will exit from being just a data and algorithm processing box and interact in the physical world directly by using robots or other physical interfaces. AI will be getting Skeletons!
The bottom line is that with embodied AI, it will be able to learn its life through interaction with the world experiencing things firsthand. The potential for this to take AI really up a notch in terms of intelligence/creativity is enormous.
Continual learning: one of the most exciting aspects of embodied AI is this. The idea is that AI can learn and change, on the fly by interacting with a living physical world.
It will not learn from a static dataset or a set, of code that has to be updated.
So, AI is going to learn from each new experience and every new face, which makes it super flexible as well as adaptable. This is the difference between reading about the world in a book and experiencing the world for yourself.
Another fascinating theory says AI might work with embodied empathy.
It means when it comes to the understanding of human emotion and experiences AI will be more realistic and empathetic. So far, AI can only recognize basic feelings like happiness or sadness.
However, with embodied AI it will understand the more complicated emotions and existence that constitute being human. This has massive implications in terms of how AI is going to experience human beings and be helping them, such as mental health, and well-being.
Embodied AI also yields another intriguing concept known as “embodied ethics” The concept of AI making decisions that mimic human nature. Right now, AI ethics tend towards programming rules and boundaries for AI systems.
With embodied AI, however, it will be able to grasp ethical items on a much more naturalistic and intricate level. It can evaluate the pros and cons of different decisions to eventually make an ethical choice depending on its past experiences.
Moving forward, the list for AI is endless. Robotics, Natural Language Processing(NLP), and Automation seem to be the fields as per expert predictions. As AI develops and evolves so do industries, reshape workforce dynamics and completely redefine society.
Conclusion
AI started as a theoretical concept and then worked its way into the world at large – often so fully you’d swear it was magic. AI is a story of creativity, success and practical impact at a very large scale and this journey of AI is thrilling.
The incorporation of AI along with its responsible development, intentional ethics, and further research is important. If we take full advantage of the epochal AI gift to augment machine and human potential, the next era will be a golden age. Maybe one day humans and AI will work and think side by side.
Artificial intelligence is a triumph and type in technological evolution that changes how we live, work, and think. From nothing more than speculative philosophical inquiries to the concrete influence it exerts on contemporary industries. The evolution of AI is a testament to human curiosity and the endless potential that the digital world offers. Deep in the era of AI, the line of discovery widens further which compels us to discover, innovate for more and redefine intelligence as always getting more embedded in machines.